From local to global integration: the digital state of pharmaceutical engineering and manufacturing
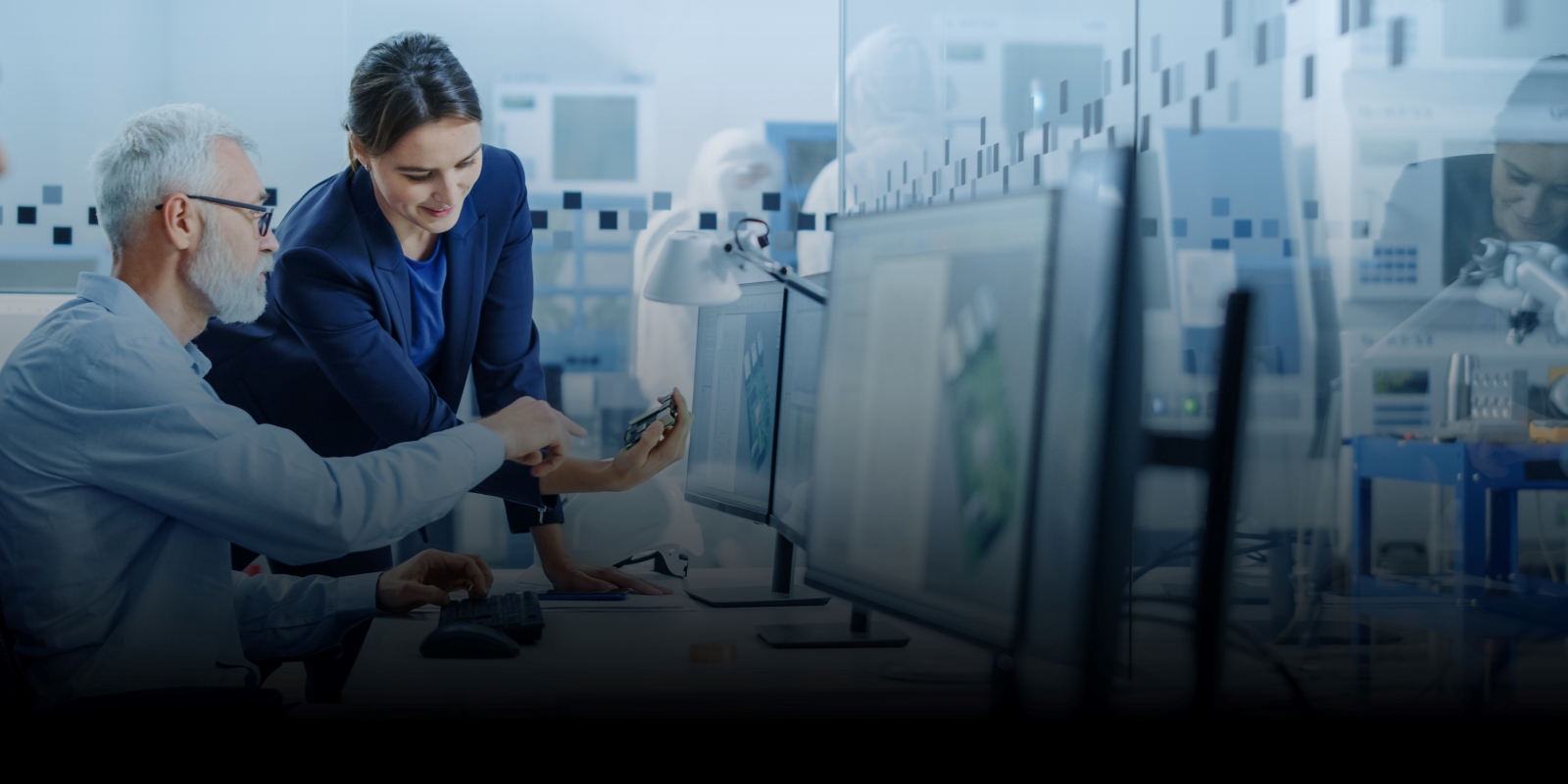
The importance of digital integration has been increasingly recognized over the past few years, but recent survey data from our Unispace Life Sciences Global Benchmark report, Forefront, gives us an even clearer picture of what trends, challenges and best practices are emerging. For this report, we surveyed 180 global industry leaders, interviewed 12 blue-chip pharma companies, and featured insights from our roundtable hosted by The International Society for Pharmaceutical Engineering (ISPE) on topics unique to pharmaceutical engineering teams. One of the six key areas we looked at was how pharmaceutical organizations are adapting to the evolving digital landscape.
How are companies using data?
The survey results demonstrated that organizations have a strong interest in interpreting data to predict scenarios and act accordingly. This is especially relevant for engineering teams and manufacturing teams who need to be able to analyze errors, successes, and trends quickly.
50% of the respondents noted that they already use data analysis to enable predictive actions, taking preventative or corrective measures before batch processes or equipment that suffer from any issues. On top of that, over the next five years we can expect an increased focus on advanced technologies such as machine learning, artificial intelligence (AI), and deep learning solutions.
We also looked at the current state of data architecture. We found that while 33% of respondents stated their data architecture is subjective and driven by existing equipment or software platforms in operation, some companies are further ahead with a site system compliance policy set in place (38%) or site-specific operating systems that provide general insight into their production processes (40%). Similarly, only 12% of respondents currently have a more mature level of compliance integration, however more than half expect to have global integration within the next five years.
Data integrity and its consequences
Data integrity is a key issue for many companies. Many note that they have no dedicated function for it or treat it as a site-based initiative only. It’s encouraging then that 46% of respondents take a risk-based approach to integrity audits. Similarly, cybersecurity remains a critical focus, with over 80% of formal programs putting responsibility with IT departments rather than engineering teams. Many engineers are aware of cybersecurity risks, but they lack knowledge when it comes to implementation strategies for security practices in their own workflows.
In the next five years, real time analytics, data analytics, and artificial intelligence/machine learning are the areas that will see the most investment. Automation is also an important development, with 60% of respondents having already invested in automating data flows, while 85% plan on beginning investments in this area within five years.
Despite all of this positive digital growth and development in the industry, there are still challenges associated with these solutions. Companies are struggling with capturing the right amount and type of data needed. And data overload is a real threat to analysts and strategists alike if companies are capturing too much data instead of the right data, leading to a lack of actionable insights. Similarly, engineers must learn how to interpret this data meaningfully to use it and make a tangible impact. Finally, organizations can become too preoccupied with overly complex data integrity requirements instead of focusing on core needs only, resulting in an inefficient distribution of resources.
Best practices
For those looking at best practices for fields becoming more digital, several ideas are being adopted by leading firms. Some respondents indicated they are outsourcing their data science function; however, more are upskilling engineers. Choosing to upskill engineers so they can effectively use data means keeping data science functions in-house for increased integrity and an investment in employee development. Similarly, instructing shop floor employees about why data integrity matters and creating a culture where data integrity is taken seriously can be easy, Other best practices include employing second witnesses when dealing with data, specifically transient data and following industry standards such as the ISPE Data Integrity Model.
Looking to the future
Overall, in an increasingly digitizing world, data analysis, integrity, and collection are quickly becoming essential elements for successful businesses. Digital development requires careful consideration when deciding which trends should be pursued and how best practices should be implemented across all levels within an organization. Not only will this help ensure companies remain competitive, but it also protects against potential risks associated with using big data technology solutions. This could include cyber security threats or inadequate quality management processes due to incomplete information capture techniques used during project execution stages. By investing in people and processes as well as technology, focusing on data integrity, and capturing the right data, companies can position themselves for success in this new digital landscape.